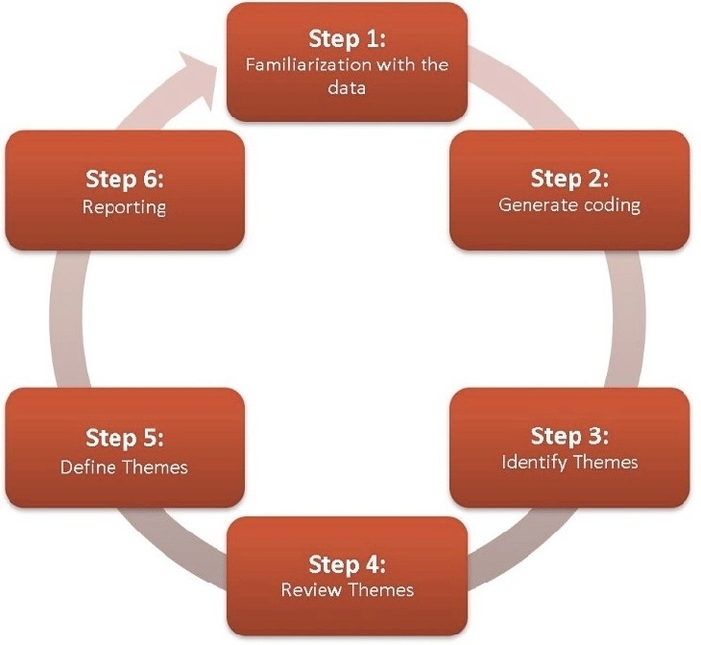
Thematic Analysis Framework is a method of analyzing qualitative data that involves identifying patterns and themes in the data. It is a flexible and widely used approach to analyzing qualitative data, and can be applied to a wide range of research questions. Thematic analysis is often used in social science research, but can also be applied to other fields such as business, education, and healthcare.
The process of conducting a thematic analysis typically involves six steps: familiarization, coding, generating themes, reviewing themes, defining and naming themes, and writing up. In the first step, the researcher becomes familiar with the data by reading through it several times. In the second step, the researcher identifies codes, or labels, that capture the meaning of the data. In the third step, the researcher generates themes by grouping together similar codes. In the fourth step, the researcher reviews the themes to ensure that they are coherent and meaningful. In the fifth step, the researcher defines and names the themes. Finally, in the sixth step, the researcher writes up the analysis, often using quotes from the data to illustrate the themes.
Thematic analysis is a flexible method that can be adapted to many different kinds of research questions. It allows researchers to identify patterns and themes in the data, and to explore the meanings and experiences of participants. However, it is also a subjective method that relies on the researcher’s judgement, and can be time-consuming and labor-intensive. Researchers must be careful to avoid confirmation bias and to reflect carefully on their own choices and interpretations.
In conclusion, Thematic Analysis Framework is a widely used method of analyzing qualitative data that involves identifying patterns and themes in the data. It is a flexible and adaptable method that can be applied to many different kinds of research questions, but it also requires careful reflection and attention to detail. Researchers who use thematic analysis must be aware of its limitations and potential biases, and must take care to ensure that their analysis is rigorous and meaningful.